by Beijing Zhongke Journal Publising Co.
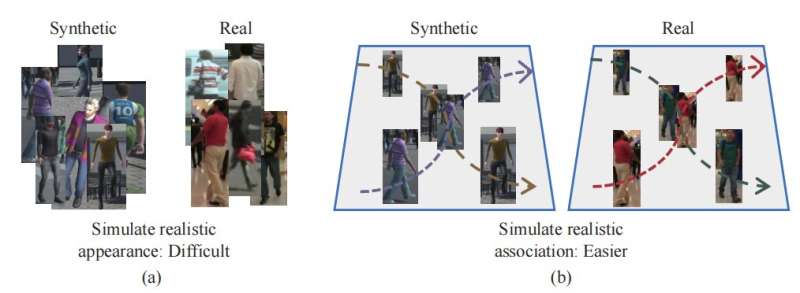
In a paper published in Machine Intelligence Research, a team of researchers study whether 3D synthetic data can replace real-world videos for association training. Specifically, they introduce a large-scale synthetic data engine named MOTX, where the motion characteristics of cameras and objects are manually configured to be similar to those of real-world datasets.
They show that, compared with real data, association knowledge obtained from synthetic data can achieve very similar performance on real-world test sets without domain adaption techniques.
Multi-object tracking (MOT) is a compound system composed of several functional components, such as detection, visual representations, and association. Association is at the final stage of the MOT pipeline and is usually viewed as the core problem, aiming to connect bounding boxes with existing tracklets.
The association module makes inferences according to appearance features, motion features, or both of them. In the community, what many solutions to the association have in common is that they are trained with real-world video data. However, there are several potential problems with this practice.
First, annotating trajectories in video frames requires expensive labor costs. This potentially limits the scale of MOT training data. Second, privacy and ethics issues constrain the usage of real-world data in human-centered tasks, for example, multiple pedestrian tracking.
In order to avoid these concerns, researchers from Australian National University and Tsinghua University investigate how to use synthetic data in MOT. They build a 3D simulation engine, MOTX, for generating videos with multiple targets, rich annotations, and controllable visual factors. Such data offer an inexpensive way to acquire large-scale data with accurate labels. With MOTX, they aim to answer two interesting questions.
The first question is whether the association knowledge learned from synthetic data work in real-world videos. A common weakness of synthetic data consists of its distribution difference with real-world data, especially regarding the image-style. In “Appearance-centered” tasks, such as re-identification and segmentation, to avoid failure in real-world test environments, models trained on synthetic data require additional training techniques, such as fine-tuning or domain adaptation on the real data.
However, association learning is different from appearance learning regarding data requirements. According to existing works, motion cues play an essential role in the association. While appearance realistic images are hard to simulate by the engine, it may be less difficult for motion cues, such as occlusion.
This study shows that on several state-of-the-art association networks, association knowledge learned from synthetic data can be well adapted to real-world scenarios without a performance drop. Specifically, researchers synthesize datasets using MOTX by manually setting key parameters (e.g., camera view) to be close to real-world training sets.
Then, when the recent association networks are trained on such synthetic videos, they achieve similar or sometimes even better tracking accuracy compared with real data training. Their ablation studies on appearance and motion features provide two suggestions.
First is the appearance-discrepancy between synthetic data and real-world data can hardly harm the association knowledge learning. Second is 3D engines can well simulate motion cues in association scenarios. The above findings can be the reason for the competitiveness of synthetic data and imply that MOT benefits more from using synthetic data than “Appearance-centered” tasks. This is a very early study of pondering the role of synthetic data in MOT.
The second question is how motion factors affect association knowledge learning. Existing datasets are mostly from the real world, such as MOT15. While these data benefit model training, that they are fixed offers us limited opportunities to understand how the system reacts to changing visual factors. For example, how does pedestrian density in the training set affect model accuracy? Can a model trained with static cameras be well deployed under moving-camera systems?
Researchers take advantage of the strong customization ability of MOTX to help answer this question. They perform empirical studies on how object-related and camera-related factors affect the learning of association knowledge. Specifically, they investigate two groups of factors.
The first group of factors is pedestrian-related factors, such as density and moving speed; The second is camera-related factors, including the camera view and camera moving state. In detail, with the proposed MOTX engine2, motion factors are abstracted with system parameters, so they can readily simulate different scenarios by simply changing these parameters, for example, setting the object velocity to 1m/s. Their results shed light on the relationship between factors in training and testing data and MOT system performance.
More information:
Yuchi Liu et al, A Study of Using Synthetic Data for Effective Association Knowledge Learning, Machine Intelligence Research (2023). DOI: 10.1007/s11633-022-1380-x
Provided by
Beijing Zhongke Journal Publising Co.
Citation:
Using synthetic data for effective association knowledge learning (2023, July 10)
retrieved 10 July 2023
from https://techxplore.com/news/2023-07-synthetic-effective-association-knowledge.html
This document is subject to copyright. Apart from any fair dealing for the purpose of private study or research, no
part may be reproduced without the written permission. The content is provided for information purposes only.
Stay connected with us on social media platform for instant update click here to join our Twitter, & Facebook
We are now on Telegram. Click here to join our channel (@TechiUpdate) and stay updated with the latest Technology headlines.
For all the latest Technology News Click Here
For the latest news and updates, follow us on Google News.