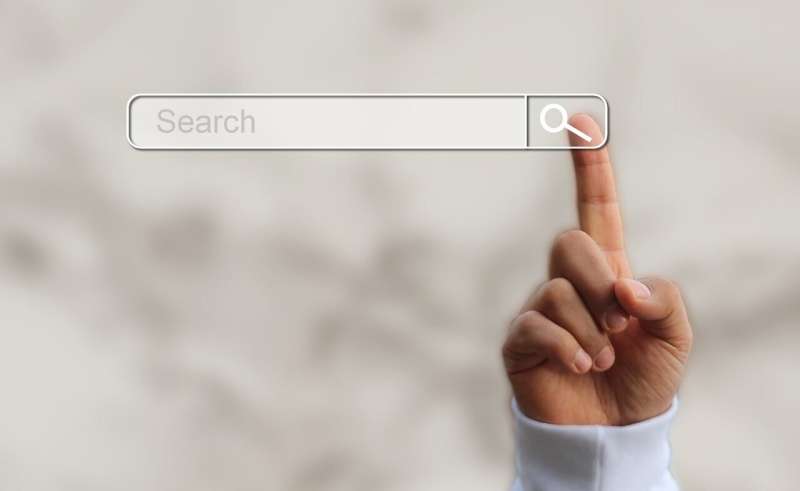
When looking for a new type of book, movie, or restaurant, your search may suggest a title or venue you’ve already purchased or experienced. This is because the artificial intelligence tools many companies rely on push users into a “filter bubble,” resulting in recommendations identical, or very similar to, what has been previously purchased.
A team of computer scientists has now devised a way to break through these search bubbles with an algorithm, Pyrorank, that draws from the natural world—mimicking interactions in an ecosystem. It does so by reducing the impact of users’ profiles and broadening recommendations that still reflect the focus of the search, producing more diverse and useful results. The work is published as a conference paper in Advances in Swarm Intelligence.
“When it comes to inspiration for solutions to computer science problems, nature is the perfect place to look,” explains Anasse Bari, a clinical associate professor at NYU’s Courant Institute of Mathematical Sciences and a co-creator of the algorithm. “Natural phenomena, such as bird flocks searching for food, show that nature can often find optimal, yet simple, solutions to address needs.”
Recommendation systems, used by Google, Netflix, and Spotify, among others, are algorithms that use data to suggest or recommend products or choices to consumers based on the users’ past purchases, search history, and demographics. However, these parameters bias search outcomes because they put users in filter bubbles.
“The traditional way recommendation systems work is by basing recommendations on the notion of similarity,” explains Bari, who leads the Courant Institute’s Predictive Analytics and AI Research Lab. “This means that you will see similar items in the choice and recommended lists based on either users similar to you or similar items you have bought. For instance, if I am an Apple product user, I will increasingly see more and more Apple products in my recommendations.”
The limitations of existing recommendation systems have become evident in striking ways. For instance, political partisans may be largely directed to news content that aligns with their pre-existing views. More significantly, recommender systems have turned up self-harm videos to susceptible individuals.
To address these concerns, Bari and his colleagues created Pyrorank, an algorithm that takes into account the content a user is seeking by capturing an array of recommendations while, at the same time, diminishing the emphasis of what the user has already purchased or interacted with. Pyrorank functions as an algorithmic “add-on” to existing recommender systems.
“This makes it highly adaptable compared to replacing the whole recommendation pipeline to promote diversification, potentially saving many engineer-hours,” explains Bari.
In testing the viability of the algorithm, the researchers compared the search outcomes generated by the Pyrorank add-on with those from traditional recommender systems by using three large datasets—MovieLens, which offers user-generated movie ratings, as well as Good Books and Goodreads, which house readers’ book ratings. They then ran a series of experiments to determine which systems created a greater diversity of recommended content while also staying true to the aims of the core recommendations.
Overall, the systems using Pyrorank generated more diverse recommendations than did existing ones, demonstrating its value in breaking through filter bubbles.
At the same time, applying Pyrorank to an existing recommendation system trades predictive accuracy—the error in predicting how much the user will like the recommendations—for increasing the diversity of the recommended items. However, this tradeoff is easily tunable for specific-use cases, the researchers say.
“You can apply a very small amount of diversity tuning and still get large gains in the range of outcomes while only marginally reducing predictive accuracy,” explains Bari. “Each case will be different, depending on the context of the recommendation system. While there is some give-and-take between diversity and accuracy, recommender systems can be calibrated to bolster the heterogeneity of search outcomes while minimizing the loss of accuracy.”
“Successful recommender systems need to be able to recognize and mitigate the biases for a user, leading to more effective recommendations and long-term health of these platforms,” he adds. “Devising algorithms to prioritize recommendation and search diversification is an important step in combating the negative effects and limitations of existing recommender systems.”
More information:
Doruk Kilitcioglu et al, Pyrorank: A Novel Nature-Inspired Algorithm to Promote Diversity in Recommender Systems, Advances in Swarm Intelligence (2023). DOI: 10.1007/978-3-031-36625-3_12
Citation:
Researchers devise algorithm to break through ‘search bubbles’ (2023, July 10)
retrieved 10 July 2023
from https://techxplore.com/news/2023-07-algorithm.html
This document is subject to copyright. Apart from any fair dealing for the purpose of private study or research, no
part may be reproduced without the written permission. The content is provided for information purposes only.
Stay connected with us on social media platform for instant update click here to join our Twitter, & Facebook
We are now on Telegram. Click here to join our channel (@TechiUpdate) and stay updated with the latest Technology headlines.
For all the latest Technology News Click Here
For the latest news and updates, follow us on Google News.