
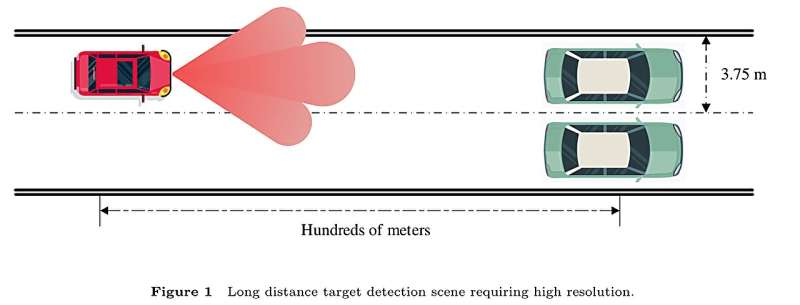
In radar perception tasks, it is necessary to estimate the direction of arrival (DOA) of the sources to achieve various applications such as target detection, tracking, and imaging. Especially for automotive millimeter-wave radars, the requirement is to achieve long-distance target detection at a high speed. Therefore, there are higher requirements for real-time performance and super-resolution ability of DOA estimation.
For such situations, deep learning-based DOA estimation methods have unique advantages. However, most existing DL-based methods use the on-grid approach, which leads to grid mismatch problems and limits their estimation accuracy and super-resolution ability.
To address the above issue, a new article published in Science China Information Sciences proposes a novel DL framework. The network consists of two parts. The first part completes a multi-label classification task, and the output result is the DOA estimates on a rough grid.
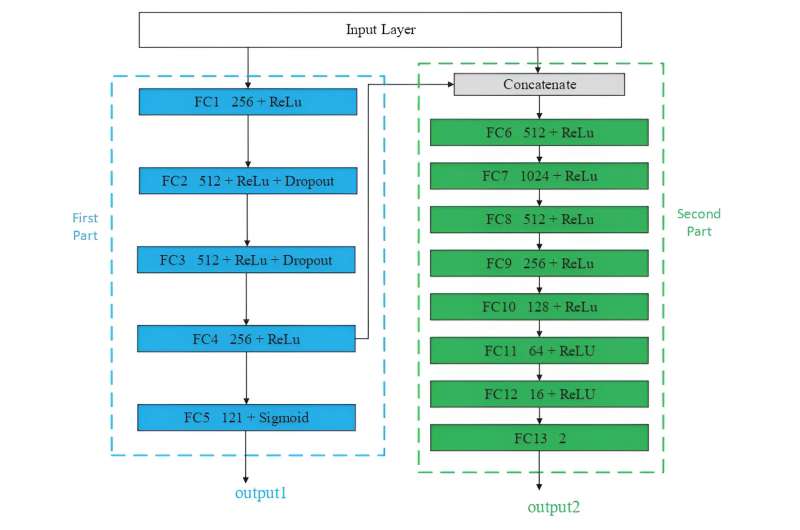
While the second part is a regression task, it further estimates the offset between the real DOAs and the grid based on the previous part. In addition, the high-resolution features of the original data are preserved through skip connection, enhancing the ability to distinguish adjacent sources. Combining the estimation results of two parts of the network, high-precision super-resolution DOA estimation results can be obtained.
The results of this study are as follows:
- The grid mismatch problem is considered in the DOA estimation problem based on DL to improve estimation accuracy.
- The estimation of the offset values between real DOAs and the grid is modeled as a regression task, ensuring that the estimation is conducted on a continuous domain.
- A novel network framework has been proposed, which can simultaneously perform on-grid DOAs estimation and grid offset estimation, and has a strong resolution ability for adjacent sources.
![DOA estimation performance on angles θ1, θ2 ∈ [−60°, 60°] with an angle interval of 3° at 17 dB SNR using T = 1000 snapshots. Credit: Science China Press Off-grid DOA estimation via deep learning framework](https://scx1.b-cdn.net/csz/news/800a/2023/off-grid-doa-estimatio-2.jpg)
The DOA estimation scheme proposed in this article has been tested on simulation and real data. A uniform linear array configuration with 12 elements is adopted and the array interelement distance equals half wavelength. The experimental results of the proposed method were compared with many traditional DOA estimation methods and other DL-based methods.
The experimental process consists of three parts. The first part is to estimate the DOAs of a series of angle pairs consisting of two sources with fixed angle intervals. These samples vary in a fixed step size within a certain angle range. From the results, it can be concluded that the proposed method can achieve stable DOA estimation with small estimation errors.
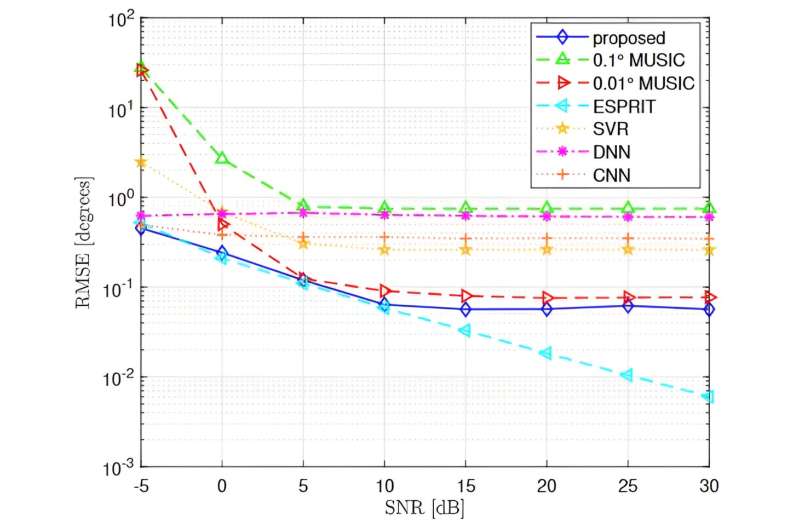
The second part of the experiment compared the statistical characteristics of various methods. Through a series of Monte Carlo simulations, the root mean square error (RMSE) performance of each method was tested and compared under different signal-to-noise ratios (SNRs), number of snapshots, and angle intervals. The experimental results show that the proposed method has good adaptability to low SNRs and small snapshots, and has strong resolution ability for sources with small angle intervals. The proposed method is significantly superior to other DL-based methods and has better performance than traditional methods in many cases.
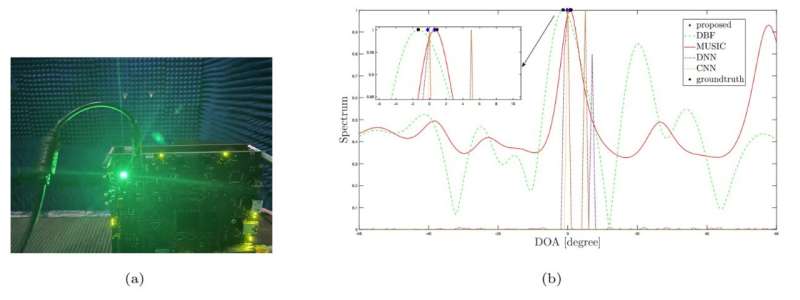
The final part of the experiment was conducted on real data, with a large number of samples collected as training data. After obtaining the trained model, a sample not included in the training set was used for testing. The DOA estimation results for real data indicate that the proposed method can accurately estimate the DOAs of two very close real targets, and is significantly superior to other methods.
More information:
Yan Huang et al, Off-Grid DOA Estimation via Deep Learning Framework, Science China Information Sciences (2023). DOI: 10.1007/s11432-022-3750-5. www.sciengine.com/SCIS/doi/10. … 07/s11432-022-3750-5
Citation:
Off-grid DOA estimation via deep learning framework (2023, September 26)
retrieved 26 September 2023
from https://techxplore.com/news/2023-09-off-grid-doa-deep-framework.html
This document is subject to copyright. Apart from any fair dealing for the purpose of private study or research, no
part may be reproduced without the written permission. The content is provided for information purposes only.
Stay connected with us on social media platform for instant update click here to join our Twitter, & Facebook
We are now on Telegram. Click here to join our channel (@TechiUpdate) and stay updated with the latest Technology headlines.
For all the latest Technology News Click Here
For the latest news and updates, follow us on Google News.