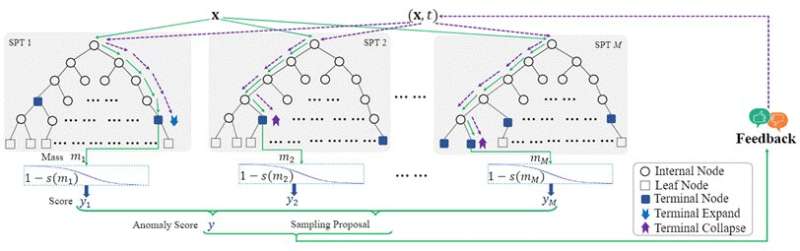
Anomaly detectors are used to distinguish differences between normal and abnormal data, which are usually implemented by evaluating and ranking the anomaly scores of each instance. A static unsupervised streaming anomaly detector is difficult to dynamically adjust anomaly score calculation.
To solve the problem, a research team led by Prof. Zhiwen Yu published their new research in Frontiers of Computer Science.
The team proposed a human-machine interactive streaming anomaly detection method, named ISPForest, which can be adaptively updated online under the guidance of human feedback. In particular, the feedback will be used to adjust the anomaly score calculation and structure of the detector, ideally attaining more accurate anomaly scores in the future.
The experimental results demonstrated that the utility of incorporating feedback can improve the performance of anomaly detectors with a few human efforts.
In the research, they analyze the anomaly detection principle of the space partitioning forest model. To improve the original anomaly detector, they add the human feedback mechanism for the detection result during the streaming anomaly detection process. First, in the light of the relationship between forest structure and anomaly score calculation, they construct regional likelihood function and instance likelihood function, respectively, to depict the consistency of the detection results and the human feedback.
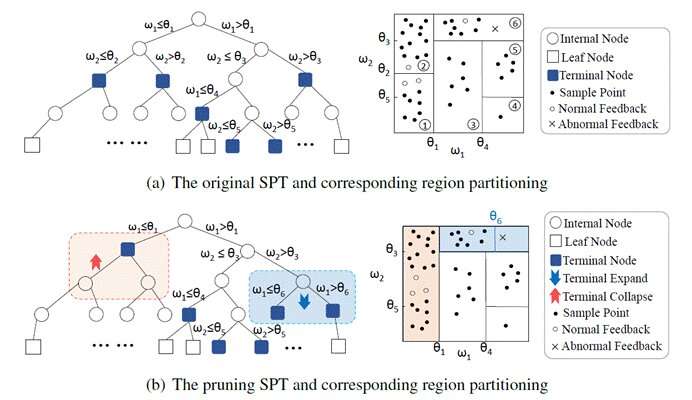
Then, the parameters and structures of the original anomaly detector are adjusted timely according to the gradient decrease process following the principle of maximum likelihood estimation. Finally, an uncertainty function of the detection results is designed to control the frequency of human-machine interaction.
Experimental results on the effects of feedback reveal that combining anomaly detectors with human feedback is meaningful for adapting to a dynamic environment, and the performance of the detector is improved promptly with a small increase in labor costs. Future work can consider the extension of the method and explore the time-series anomaly detection under the feedback mechanism.
More information:
Qingyang Li et al, Human-machine interactive streaming anomaly detection by online self-adaptive forest, Frontiers of Computer Science (2022). DOI: 10.1007/s11704-022-1270-y
Provided by
Higher Education Press
Citation:
An online adaptive model for streaming anomaly detection based on human-machine cooperation (2023, May 5)
retrieved 5 May 2023
from https://techxplore.com/news/2023-05-online-streaming-anomaly-based-human-machine.html
This document is subject to copyright. Apart from any fair dealing for the purpose of private study or research, no
part may be reproduced without the written permission. The content is provided for information purposes only.
Stay connected with us on social media platform for instant update click here to join our Twitter, & Facebook
We are now on Telegram. Click here to join our channel (@TechiUpdate) and stay updated with the latest Technology headlines.
For all the latest Technology News Click Here
For the latest news and updates, follow us on Google News.