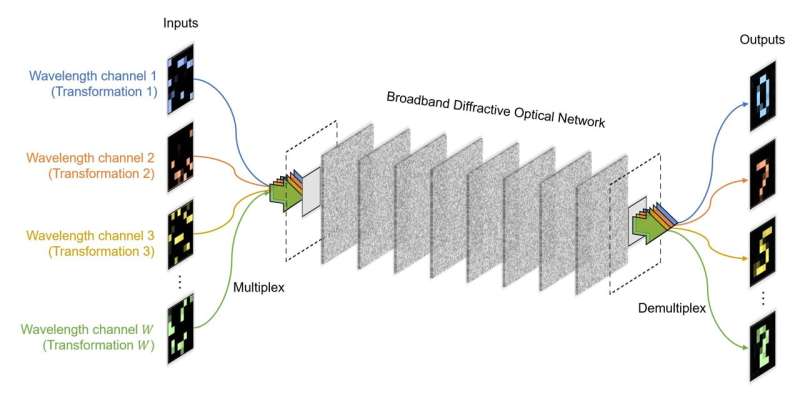
Computing using light can potentially provide lower latency and reduced power consumption, benefiting from the parallelism that optical systems have. For example, a single optical processor can execute many distinct computational tasks simultaneously, such as the parallel computing of many different transformations, which could be valuable for numerous applications, including the acceleration of machine learning-based inference.
A new research paper, published in Advanced Photonics, demonstrated the feasibility of massively parallel optical computing by employing a wavelength multiplexing scheme. In their publication, researchers from the University of California, Los Angeles (UCLA) reported a wavelength-multiplexed diffractive optical processor that enables the simultaneous computation of hundreds of different complex-valued linear transformations through different wavelength channels.
Designed using deep learning tools, this diffractive optical processor consists of structured diffractive surfaces, made of passive transmissive materials. In this optical processor, a pre-determined group of discrete wavelengths encodes the input and output information. Each wavelength is dedicated to a unique target function or complex-valued linear transformation. Following the deep learning-based design phase, this processor can be fabricated using 3D printing or photolithography and then assembled to physically form an optical processor, which can simultaneously perform a large group of target transformations between its input and output.
These target transforms can be specifically assigned for distinct functions, including, for example, image classification, segmentation, encryption, and reconstruction, or they can be dedicated to computing different convolutional filter operations or fully connected layers in a neural network. By virtue of this unique wavelength multiplexed design, all these linear transforms or desired functions are executed simultaneously at the speed of light, where each desired function is assigned to a unique wavelength, allowing the broadband optical processor to compute with extreme throughput and parallelism.
This broadband diffractive processor design does not require any wavelength-selective elements such as spectral or color filters, and is compatible with a wide range of materials with different dispersion properties. The UCLA researchers believe that this platform and the underlying concepts can be used to develop high-performance optical processors that operate at different parts of the electromagnetic spectrum, including the visible and infrared wavelengths.
In addition, due to its capability to directly process the input spectral information, the reported framework will also inspire the development of multicolor and hyperspectral machine vision systems that perform statistical inference based on the spatial and spectral information of the input objects, which might find applications in biomedical imaging and sensing for the detection and specific imaging of substances with unique spectral characteristics.
Jingxi Li et al, Massively parallel universal linear transformations using a wavelength-multiplexed diffractive optical network, Advanced Photonics (2023). DOI: 10.1117/1.AP.5.1.016003
Citation:
Wavelength-multiplexed diffractive optical processor computes hundreds of linear transformations in parallel (2023, January 10)
retrieved 10 January 2023
from https://techxplore.com/news/2023-01-wavelength-multiplexed-diffractive-optical-processor-hundreds.html
This document is subject to copyright. Apart from any fair dealing for the purpose of private study or research, no
part may be reproduced without the written permission. The content is provided for information purposes only.
Stay connected with us on social media platform for instant update click here to join our Twitter, & Facebook
We are now on Telegram. Click here to join our channel (@TechiUpdate) and stay updated with the latest Technology headlines.
For all the latest Technology News Click Here
For the latest news and updates, follow us on Google News.